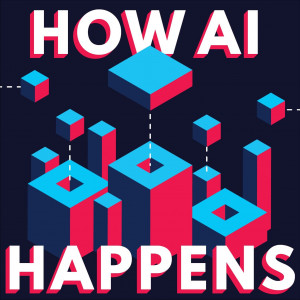
How AI Happens is a podcast featuring experts and practitioners explaining their work at the cutting edge of Artificial Intelligence. Tune in to hear AI Researchers, Data Scientists, ML Engineers, and the leaders of today’s most exciting AI companies explain the newest and most challenging facets of their field. Powered by Sama.
Mar 20, 2025
Mica shares the methods behind Augury’s fault testing processes, why they use the highest quality data available, how in-house experts help them filter their data reliably, and their approach to communicating with customers. Our conversation also explores the balance between edge computing and cloud computing, and why both are necessary for optimal performance and monitoring.Key Points From This Episode:Mica’s journey from studying physics at the Weizmann Institute to her current role at Augury.How her background in physics and neuroscience inform her work in AI.Why physicists are drawn to AI and data science; how scientists follow their curiosity.Mica’s responsibilities in her role as algorithms team lead at Augury.How they develop algorithms and test for faults; why this requires the highest quality data.Understanding the role of their in-house expert vibration analysts.The importance of domain expertise in labeling and annotating data.Finding the balance between manual and automated processes in data labeling.How to communicate with customers and present metrics that matter to them.Augury’s use of edge and cloud computing for optimal performance and monitoring.Quotes:“We look for better ways to adjust our algorithms and also develop new ones for all kinds of faults that could happen in the machines catching events that are trickier to catch, and for that we need highest quality data.” — Mica Rubinson [0:08:20]“At Aubrey, we have internal vibration analysts that are experts in their field. They go through very rigorous training process. There are international standards to how you do vibration analysis, and we have them in-house.” — Mica Rubinson [0:09:07]“[It’s] really helpful for us to have [these] in-house experts. We have massive amounts of records – signal recordings from 10 years of machine monitoring. Thanks to these experts [in] labeling, we can filter out a lot of noisy parts of this data.” — Mica Rubinson [0:10:32]“We quantify [our services] for the customer as their ROI [and] how much they saved by using Augury. You had this [issue, and] we avoided this downtime. [We show] how much does it translates eventually [into] money that you saved.” — Mica Rubinson [0:22:28]Links Mentioned in Today’s Episode:Mica Rubinson on LinkedInMica Rubinson on ResearchGateAuguryWeizmann Institute of ScienceHow AI HappensSama
00:31:47
Feb 28, 2025
Srini highlights the importance of integrating these agents into real-world applications, enhancing productivity and user experiences across industries. Srini also delves into the challenges of building reliable, ethical, and secure AI systems while fostering developer innovation. His insights offer a roadmap for harnessing advanced agents to drive meaningful technological progress. Don’t miss this informative conversation. Key Points From This Episode:Introducing today’s guest, Srini Iragavarapu, a leader at AWS. His thoughts on how Agentic and AI are intersecting today. The state of the union of agents in the world and at AWS.How AWS is leveraging agents to build specific tasks for customers.Two mechanisms that software agents use to operate.Understanding the reasoning capabilities of large foundational models.How AWS makes use of a test agent. Qdeveloper’s instantaneous conversational capabilities.Bringing different options to the customers as a long-term strategy.Three layers at which AWS is innovating today.Why the end user is ultimately the person who benefits. Quotes:“Think of it as an iterative way of solving a problem rather than just calling a single API and coming back: that’s in a nutshell how generative AI and the foundation models are working with reasoning capabilities.” — Srini Iragavarapu [0:03:04]“The models are becoming more powerful and more available, faster, a lot more dependable.” — Srini Iragavarapu [0:29:57]Links Mentioned in Today’s Episode:Srini Iragavarapu on LinkedInHow AI HappensSama
00:32:47
Feb 21, 2025
We explore the current trends of AI-based solutions in retail, what has driven its adoption in the industry, and how AI-based customer service technology has improved over time. We also discuss the correct mix of technology and humans, the importance of establishing boundaries for AI, and why it won't replace humans but will augment workflow. Hear examples of AI retail success stories, what companies got AI wrong, and the reasons behind the wins and failures. Gain insights into the value of copilots, business strategies to avoid investing in ineffective AI solutions, and much more. Tune in now!Key Points From This Episode:Learn about Lisa and Mika's backgrounds in retail technology and AI-based solutions. Hear how AI has become more accessible to businesses beyond the typical tech giants.Explore how AI-powered chatbots and copilots have evolved to improve customer service.The Coca-Cola AI ad controversy and why oversight on AI-generated content is vital.Discover the innovative and exciting ways AI can be leveraged in the retail industry.AI success stories: Target’s AI copilot for employees and Nordstrom’s personalization tool.How AI is making the return process more efficient and improving inventory management.Uncover the multimodal connections of AI and how it will enhance customer personalization.Important considerations for businesses regarding the adoption of AI and the pitfalls to avoid.Quotes:“I think [the evolution] in terms of accessibility to AI-solutions for people who don't have the massive IT departments and massive data analytics departments is really remarkable.” — Mika Yamamoto [0:04:25]“Whether it's generative AI for creative or content or whatever, it's not going to replace humans. It's going to augment our workflows.” — Lisa Avvocato [0:10:46]“Retail is actually one of the fastest adopting industries out there [of] AI.” — Mika Yamamoto [0:14:17]“Having conversations with peers, I think, is absolutely invaluable to figure out what's hype and what's reality [regarding AI].” — Mika Yamamoto [0:30:19]Links Mentioned in Today’s Episode:Lisa Avvocato on LinkedInMika Yamamoto on LinkedInFreshworksThe Coca‑Cola CompanyHow AI HappensSama
00:32:11
Jan 30, 2025
We hear about Nitzan’s AI expertise, motivation for joining eBay, and approach to implementing AI into eBay's business model. Gain insights into the impacts of centralizing and federating AI, leveraging generative AI to create personalized content, and why patience is essential to AI development. We also unpack eBay's approach to LLM development, tailoring AI tools for eBay sellers, the pitfalls of generic marketing content, and the future of AI in retail. Join us to discover how AI is revolutionizing e-commerce and disrupting the retail sector with Nitzan Mekel-Bobrov! Key Points From This Episode:Nitzan's career experience, his interest in sustainability, and his sneaker collection.Why he decided to begin a career at eBay and his role at the company.His approach to aligning the implementation of AI with eBay's overall strategy.How he identifies the components of eBay's business model that will benefit from AI.What makes eBay highly suitable for the implementation of AI tools.Challenges of using generative AI models to create personalized content for users.Why experimentation is vital to the AI development and implementation process.Aspects of the user experience that Nitzan uses to train and develop eBay's LLMs.The potential of knowledge graphs to uncover the complexity of user behavior.Reasons that the unstructured nature of eBay's data is fundamental to its business model.Incorporating a seller's style into AI tools to avoid creating generic marketing material.Details about Nitzan’s team and their diverse array of expertise.Final takeaways and how companies can ensure they survive the AI transition. Quotes:“It’s tricky to balance the short-term wins with the long-term transformation.” — Nitzan Mekel-Bobrov [0:06:50]“An experiment is only a failure if you haven’t learned anything yourself and – generated institutional knowledge from it.” — Nitzan Mekel-Bobrov [0:09:36]“What's nice about [eBay's] business model — is that our incentive is to enable each seller to maintain their own uniqueness.” — Nitzan Mekel-Bobrov [0:27:33]“The companies that will thrive in this AI transformation are the ones that can figure out how to marry parts of their current culture and what all of their talent brings with what the AI delivers.” — Nitzan Mekel-Bobrov [0:33:58]Links Mentioned in Today’s Episode:Nitzan Mekel-Bobrov on LinkedIneBayHow AI HappensSama
00:33:00
Jan 24, 2025
Satya unpacks how Unilever utilizes its database to inform its models and how to determine the right amount of data needed to solve complex problems. Dr. Wattamwar explains why contextual problem-solving is vital, the notion of time constraints in data science, the system point of view of modeling, and how Unilever incorporates AI into its models. Gain insights into how AI can increase operational efficiency, exciting trends in the AI space, how AI makes experimentation accessible, and more! Tune in to learn about the power of data science and AI with Dr. Satyajit Wattamwar. Key Points From This Episode:Background on Dr. Wattamwar, his PhD research, and data science expertise.Unpacking some of the commonalities between data science and physics. Why the outcome of using significantly large data sets depends on the situation. The minimum amount of data needed to make meaningful and quality models.Examples of the common mistakes and pitfalls that data scientists make.How Unilever works with partner organizations to integrate AI into its models.Ways that Dr. Wattamwar uses AI-based tools to increase his productivity.The difference between using AI for innovation versus operational efficiency.Insight into the shifting data science landscape and advice for budding data scientists.Quotes:“Around – 30 or 40 years ago, people started realizing the importance of data-driven modeling because you can never capture physics perfectly in an equation.” — Dr. Satyajit Wattamwar [0:03:10]“Having large volumes of data which are less related with each other is a different thing than a large volume of data for one problem.” — Dr. Satyajit Wattamwar [0:09:12]“More data [does] not always lead to good quality models. Unless it is for the same use-case.” — Dr. Satyajit Wattamwar [0:11:56]“If somebody is looking [to] grow in their career ladder, then it's not about one's own interest.” — Dr. Satyajit Wattamwar [0:24:07]Links Mentioned in Today’s Episode:Dr. Satyajit Wattamwar on LinkedInUnileverHow AI HappensSama
00:25:05
Dec 30, 2024
Jing explains how Vanguard uses machine learning and reinforcement learning to deliver personalized "nudges," helping investors make smarter financial decisions. Jing dives into the importance of aligning AI efforts with Vanguard’s mission and discusses generative AI’s potential for boosting employee productivity while improving customer experiences. She also reveals how generative AI is poised to play a key role in transforming the company's future, all while maintaining strict data privacy standards.Key Points From This Episode:Jing Wang’s time at Fermilab and the research behind her PhD in high-energy physics.What she misses most about academia and what led to her current role at Vanguard.How she aligns her team’s AI strategy with Vanguard’s business goals.Ways they are utilizing AI for nudging investors to make better decisions.Their process for delivering highly personalized recommendations for any given investor.Steps that ensure they adhere to finance industry regulations with their AI tools.The role of reinforcement learning and their ‘next best action’ models in personalization.Their approach to determining the best use of their datasets while protecting privacy.Vanguard’s plans for generative AI, from internal productivity to serving clients.How Jing stays abreast of all the latest developments in physics.Quotes:“We make sure all our AI work is aligned with [Vanguard’s] four pillars to deliver business impact.” — Jing Wang [0:08:56]“We found those simple nudges have tremendous power in terms of guiding the investors to adopt the right things. And this year, we started to use a machine learning model to actually personalize those nudges.” — Jing Wang [0:19:39]“Ultimately, we see that generative AI could help us to build more differentiated products. – We want to have AI be able to train language models [to have] much more of a Vanguard mindset.” — Jing Wang [0:29:22]Links Mentioned in Today’s Episode:Jing Wang on LinkedInVanguardFermilabHow AI HappensSama
00:35:09
Dec 23, 2024
Key Points From This Episode:Ram Venkatesh describes his career journey to founding Sema4.ai. The pain points he was trying to ease with Sema4.ai.How our general approach to big data is becoming more streamlined, albeit rather slowly. The ins and outs of Sema4.ai and how it serves its clients. What Ram means by “agent” and “agent agency” when referring to machine learning copilots.The difference between writing a program to execute versus an agent reasoning with it. Understanding the contextual work training method for agents. The relationship between an LLM and an agent and the risks of training LLMs on agent data.Exploring the next generation of LLM training protocols in the hopes of improving efficiency. The requirements of an LLM if you’re not training it and unpacking modality improvements. Why agent input and feedback are major disruptions to SaaS and beyond. Our guest shares his hopes for the future of AI. Quotes:“I’ve spent the last 30 years in data. So, if there’s a database out there, whether it’s relational or object or XML or JSON, I’ve done something unspeakable to it at some point.” — @ramvzz [0:01:46]“As people are getting more experienced with how they could apply GenAI to solve their problems, then they’re realizing that they do need to organize their data and that data is really important.” — @ramvzz [0:18:58]“Following the technology and where it can go, there’s a lot of fun to be had with that.” — @ramvzz [0:23:29]“Now that we can see how software development itself is evolving, I think that 12-year-old me would’ve built so many more cooler things than I did with all the tech that’s out here now.” — @ramvzz [0:29:14]Links Mentioned in Today’s Episode:Ram Venkatesh on LinkedInRam Venkatesh on XSema4.aiClouderaHow AI HappensSama
00:30:17
Dec 18, 2024
Pascal & Yannick delve into the kind of human involvement SAM-2 needs before discussing the use cases it enables. Hear all about the importance of having realistic expectations of AI, what the cost of SAM-2 looks like, and the the importance of humans in LLMs.Key Points From This Episode:Introducing Pascal Jauffret and Yannick Donnelly to the show.Our guests explain what the SAM-2 model is. A description of what getting information from video entails.What made our guests interested in researching SAM-2. A few things that stand out about this tool. The level of human involvement that SAM-2 needs. Some of the use cases they see SAM-2 enabling. Whether manually annotating is easier than simply validating data. The importance of setting realistic expectations of what AI can do. When LLM models work best, according to our experts.A discussion about the cost of the models at the moment. Why humans are so important in coaching people to use models. What we can expect from Sama in the near future. Quotes:“We’re kind of shifting towards more of a validation period than just annotating from scratch.” — Yannick Donnelly [0:22:01]“Models have their place but they need to be evaluated.” — Yannick Donnelly [0:25:16]“You’re never just using a model for the sake of using a model. You’re trying to solve something and you’re trying to improve a business metric.” — Pascal Jauffret [0:32:59]“We really shouldn’t underestimate the human aspect of using models.” — Pascal Jauffret [0:40:08]Links Mentioned in Today’s Episode:Pascal Jauffret on LinkedInYannick Donnelly on LinkedInHow AI HappensSama
00:49:53
Dec 16, 2024
Today we are joined by Siddhika Nevrekar, an experienced product leader passionate about solving complex problems in ML by bringing people and products together in an environment of trust. We unpack the state of free computing, the challenges of training AI models for edge, what Siddhika hopes to achieve in her role at Qualcomm, and her methods for solving common industry problems that developers face.Key Points From This Episode:Siddhika Nevrekar walks us through her career pivot from cloud to edge computing. Why she’s passionate about overcoming her fears and achieving the impossible. Increasing compute on edge devices versus developing more efficient AI models.Siddhika explains what makes Apple a truly unique company. The original inspirations for edge computing and how the conversation has evolved. Unpacking the current state of free computing and what may happen in the near future. The challenges of training AI models for edge. Exploring Siddhika’s role at Qualcomm and what she hopes to achieve. Diving deeper into her process for achieving her goals. Common industry challenges that developers are facing and her methods for solving themQuotes:“Ultimately, we are constrained with the size of the device. It’s all physics. How much can you compress a small little chip to do what hundreds and thousands of chips can do which you can stack up in a cloud? Can you actually replicate that experience on the device?” — @siddhika_ “By the time I left Apple, we had 1000-plus [AI] models running on devices and 10,000 applications that were powered by AI on the device, exclusively on the device. Which means the model is entirely on the device and is not going into the cloud. To me, that was the realization that now the moment has arrived where something magical is going to start happening with AI and ML.” — @siddhika_ Links Mentioned in Today’s Episode:Siddhika Nevrekar on LinkedInSiddhika Nevrekar on XQualcomm AI HubHow AI HappensSama
00:32:57
Dec 03, 2024
Today we are joined by Developer Advocate at Block, Rizel Scarlett, who is here to explain how to bridge the gap between the technical and non-technical aspects of a business. We also learn about AI hallucinations and how Rizel and Block approach this particular pain point, the burdens of responsibility of AI users, why it’s important to make AI tools accessible to all, and the ins and outs of G{Code} House – a learning community for Indigenous and women of color in tech. To end, Rizel explains what needs to be done to break down barriers to entry for the G{Code} population in tech, and she describes the ideal relationship between a developer advocate and the technical arm of a business. Key Points From This Episode:Rizel Scarlett describes the role and responsibilities of a developer advocate. Her role in getting others to understand how GitHub Copilot should be used. Exploring her ongoing projects and current duties at Block. How the conversation around AI copilot tools has shifted in the last 18 months. The importance of objection handling and why companies must pay more attention to it. AI hallucinations and Rizel’s advice for approaching this particular pain point. Why “I don’t know” should be encouraged as a response from AI companions, not shunned. Taking a closer look at how Block addresses AI hallucinations. The burdens of responsibility of users of AI, and the need to democratize access to AI tools. Unpacking G{Code} House and Rizel’s working relationship with this learning community.Understanding what prevents Indigenous and women of color from having careers in tech.The ideal relationship between a developer advocate and the technical arm of a business. Quotes:“Every company is embedding AI into their product someway somehow, so it’s being more embraced.” — @blackgirlbytes [0:11:37]“I always respect someone that’s like, ‘I don’t know, but this is the closest I can get to it.’” — @blackgirlbytes [0:15:25]“With AI tools, when you’re more specific, the results are more refined.” — @blackgirlbytes [0:16:29]Links Mentioned in Today’s Episode:Rizel ScarlettRizel Scarlett on LinkedInRizel Scarlett on InstagramRizel Scarlett on XBlockGooseGitHubGitHub CopilotG{Code} HouseHow AI HappensSama
00:28:10
Nov 21, 2024
Key Points From This Episode:Drew and his co-founders’ background working together at RJ Metrics.The lack of existing data solutions for Amazon Redshift and how they started dbt Labs.Initial adoption of dbt Labs and why it was so well-received from the very beginning.The concept of a semantic layer and how dbt Labs uses it in conjunction with LLMs.Drew’s insights on a recent paper by Apple on the limitations of LLMs’ reasoning.Unpacking examples where LLMs struggle with specific questions, like math problems.The importance of thoughtful prompt engineering and application design with LLMs.What is needed to maximize the utility of LLMs in enterprise settings.How understanding the specific use case can help you get better results from LLMs.What developers can do to constrain the search space and provide better output.Why Drew believes prompt engineering will become less important for the average user.The exciting potential of vector embeddings and the ongoing evolution of LLMs.Quotes:“Our observation was [that] there needs to be some sort of way to prepare and curate data sets inside of a cloud data warehouse. And there was nothing out there that could do that on [Amazon] Redshift, so we set out to build it.” — Drew Banin [0:02:18]“One of the things we're thinking a ton about today is how AI and the semantic layer intersect.” — Drew Banin [0:08:49]“I don't fundamentally think that LLMs are reasoning in the way that human beings reason.” — Drew Banin [0:15:36]“My belief is that prompt engineering will – become less important – over time for most use cases. I just think that there are enough people that are not well versed in this skill that the people building LLMs will work really hard to solve that problem.” — Drew Banin [0:23:06]Links Mentioned in Today’s Episode: Understanding the Limitations of Mathematical Reasoning in Large Language ModelsDrew Banin on LinkedIndbt LabsHow AI HappensSama
00:28:02
Oct 31, 2024
In this episode, you’ll hear about Meeri's incredible career, insights from the recent AI Pact conference she attended, her company's involvement, and how we can articulate the reality of holding companies accountable to AI governance practices. We discuss how to know if you have an AI problem, what makes third-party generative AI more risky, and so much more! Meeri even shares how she thinks the Use AI Act will impact AI companies and what companies can do to take stock of their risk factors and ensure that they are building responsibly. You don’t want to miss this one, so be sure to tune in now!Key Points From This Episode:Insights from the AI Pact conference. The reality of holding AI companies accountable. What inspired her to start Saidot to offer solutions for AI transparency and accountability.How Meeri assesses companies and their organizational culture. What makes generative AI more risky than other forms of machine learning. Reasons that use-related risks are the most common sources of AI risks.Meeri’s thoughts on the impact of the Use AI Act in the EU. Quotes:“It’s best to work with companies who know that they already have a problem.” — @meerihaataja [0:09:58]“Third-party risks are way bigger in the context of [generative AI].” — @meerihaataja [0:14:22]“Use and use-context-related risks are the major source of risks.” — @meerihaataja [0:17:56]“Risk is fine if it’s on an acceptable level. That’s what governance seeks to do.” — @meerihaataja [0:21:17]Links Mentioned in Today’s Episode:SaidotMeeri Haataja on LinkedInMeeri Haataja on InstagramMeeri Haataja on XHow AI HappensSama
00:25:18
Oct 18, 2024
In this episode, Dr. Zoldi offers insight into the transformative potential of blockchain for ensuring transparency in AI development, the critical need for explainability over mere predictive power, and how FICO maintains trust in its AI systems through rigorous model development standards. We also delve into the essential integration of data science and software engineering teams, emphasizing that collaboration from the outset is key to operationalizing AI effectively. Key Points From This Episode:How Scott integrates his role as an inventor with his duties as FICO CAO.Why he believes that mindshare is an essential leadership quality.What sparked his interest in responsible AI as a physicist.The shifting demographics of those who develop machine learning models.Insight into the use of blockchain to advance responsible AI.How FICO uses blockchain to ensure auditable ML decision-making.Operationalizing AI and the typical mistakes companies make in the process.The value of integrating data science and software engineering teams from the start.A fear-free perspective on what Scott finds so uniquely exciting about AI.Quotes:“I have to stay ahead of where the industry is moving and plot out the directions for FICO in terms of where AI and machine learning is going – [Being an inventor is critical for] being effective as a chief analytics officer.” — @ScottZoldi [0:01:53]“[AI and machine learning] is software like any other type of software. It's just software that learns by itself and, therefore, we need [stricter] levels of control.” — @ScottZoldi [0:23:59]“Data scientists and AI scientists need to have partners in software engineering. That's probably the number one reason why [companies fail during the operationalization process].” — @ScottZoldi [0:29:02]Links Mentioned in Today’s Episode:FICODr. Scott ZoldiDr. Scott Zoldi on LinkedInDr. Scott Zoldi on XFICO Falcon Fraud ManagerHow AI HappensSama
00:33:39
Oct 10, 2024
Jay breaks down the critical role of software optimizations and how they drive performance gains in AI, highlighting the importance of reducing inefficiencies in hardware. He also discusses the long-term vision for Lemurian Labs and the broader future of AI, pointing to the potential breakthroughs that could redefine industries and accelerate innovation, plus a whole lot more. Key Points From This Episode:Jay’s diverse professional background and his attraction to solving unsolvable problems.How his unfinished business in robotics led him to his current work at Lemurian Labs.What he has learned from being CEO and the biggest obstacles he has had to overcome.Why he believes engineers with a problem-solving mindset can be effective CEOs.Lemurian Labs: making AI computing more efficient, affordable, and environmentally friendly.The critical role of software in increasing AI efficiency.Some of the biggest challenges in programming GPUs.Why better software is needed to optimize the use of hardware.Common inefficiencies in AI development and how to solve them.Reflections on the future of Lemurian Labs and AI more broadly.Quotes:“Every single problem I've tried to pick up has been one that – most people have considered as being almost impossible. There’s something appealing about that.” — Jay Dawani [0:02:58]“No matter how good of an idea you put out into the world, most people don't have the motivation to go and solve it. You have to have an insane amount of belief and optimism that this problem is solvable, regardless of how much time it's going to take.” — Jay Dawani [0:07:14]“If the world's just betting on one company, then the amount of compute you can have available is pretty limited. But if there's a lot of different kinds of compute that are slightly optimized with different resources, making them accessible allows us to get there faster.” — Jay Dawani [0:19:36]“Basically what we're trying to do [at Lemurian Labs] is make it easy for programmers to get [the best] performance out of any hardware.” — Jay Dawani [0:20:57]Links Mentioned in Today’s Episode:Jay Dawani on LinkedInLemurian LabsHow AI HappensSama
00:29:27
Sep 30, 2024
Melissa explains the importance of giving developers the choice of working with open source or proprietary options, experimenting with flexible application models, and choosing the size of your model according to the use case you have in mind. Discussing the democratization of technology, we explore common challenges in the context of AI including the potential of generative AI versus the challenge of its implementation, where true innovation lies, and what Melissa is most excited about seeing in the future.Key Points From This Episode:An introduction to Melissa Evers, Vice President and General Manager of Strategy and Execution at Intel Corporation.More on the communities she has played a leadership role in.Why open source governance is not an oxymoron and why it is critical.The hard work that goes on behind the scenes at open source.What to strive for when building a healthy open source community.Intel’s perspective on the importance of open source and open AI.Enabling developer choices about open source or proprietary options.Growing awareness around building architecture around the freedom of choice.Identifying that a model is a bad choice or lacking in accuracy.Thinking critically about future-proofing yourself with regard to model choice. Opportunities for large and smaller models.Finding the perfect intersection between value delivery, value creation, and cost. Common challenges in the context of AI, including the potential of generative AI and its implementation.Why there is such a commonality of use cases in the realm of generative AI.Where true innovation and value lies even though there may be commonality in use cases.Examples of creative uses of generative AI; retail, compound AI systems, manufacturing, and more.Understanding that innovation in this area is still in its early development stages. How Wardley Mapping can support an understanding of scale. What she is most excited about for the future of AI: Rapid learning in healthcare. Quotes:“One of the things that is true about software in general is that the role that open source plays within the ecosystem has dramatically shifted and accelerated technology development at large.” — @melisevers [0:03:02]“It’s important for all citizens of the open source community, corporate or not, to understand and own their responsibilities with regard to the hard work of driving the technology forward.” — @melisevers [0:05:18]“We believe that innovation is best served when folks have the tools at their disposal on which to innovate.” — @melisevers [0:09:38]“I think the focus for open source broadly should be on the elements that are going to be commodified.” — @melisevers [0:25:04]Links Mentioned in Today’s Episode:Melissa Evers on LinkedInMelissa Evers on XIntel Corporation
00:35:05
Sep 27, 2024
VP of AI and ML at Synopsys, Thomas Andersen joins us to discuss designing AI chips. Tuning in, you’ll hear all about our guest’s illustrious career, how he became interested in technology, tech in East Germany, what it was like growing up there, and so much more! We delve into his company, Synopsys, and the chips they build before discussing his role in building algorithms. Key Points From This Episode:A warm welcome to today’s guest, Thomas Andersen. How he got into the tech world and his experience growing up in East Germany. The cost of Compute AI coming down at the same time the demand is going up. Thomas tells us about Synopsys and what goes into building their chips. Other traditional software companies that are now designing their own AI chips. What Thomas’ role looks like in machine learning and building AI algorithms. How the constantly changing rules of AI chip design continue to create new obstacles. Thomas tells us how they use reinforcement learning in their processes.The different applications for generative AI and why it needs good input data. Thomas’ advice for anyone wanting to get into the world of AI. Quotes:“It’s not really the technology that makes life great, it’s how you use it, and what you make of it.” — Thomas Andersen [0:07:31]“There is, of course, a lot of opportunities to use AI in chip design.” — Thomas Andersen [0:25:39]“Be bold, try as many new things [as you can, and] make sure you use the right approach for the right tasks.” — Thomas Andersen [0:40:09]Links Mentioned in Today’s Episode:Thomas Andersen on LinkedInSynopsysHow AI HappensSama
00:41:32
Sep 24, 2024
Developing AI and generative AI initiatives demands significant investment, and without delivering on customer satisfaction, these costs can be tough to justify. Today, SVP of Engineering and General Manager of Xactly India, Kandarp Desai joins us to discuss Xactly's AI initiatives and why customer satisfaction remains their top priority. Key Points From This Episode:An introduction to Kandarp and his transition from hardware to software.How he became SVP of Engineering and General Manager of Xactly India.His move to Bangalore and the expansion of Xactly’s presence in India.The rapid modernization of India as a key factor in Xactly’s growth strategy.An overview of Xactly’s AI and generative AI initiatives.Insight into the development of Xactly’s AI Copilot.Four key stakeholders served by the Xactly AI Copilot.The Xactly Extend, an enterprise platform for building custom apps.Challenges in justifying the ROI of AI initiatives.Why customer satisfaction and business outcomes are essential.How AI is overhyped in the short term and underhyped in the long term.The difficulties in quantifying the value of AI.Kandarp’s career advice to AI practitioners, from taking risks to networking.Quotes:“[Generative AI] is only useful if it drives higher customer satisfaction. Otherwise, it doesn't matter.” — Kandarp Desai [0:11:36]“Justifying the ROI of anything is hard – If you can tie any new invention back to its ROI in customer satisfaction, that can drive an easy sell across an organization.” — Kandarp Desai [0:15:35]“The whole AI trend is overhyped in the short term and underhyped long term. [It’s experienced an] oversell recently, and people are still trying to figure it out.” — Kandarp Desai [0:20:48]Links Mentioned in Today’s Episode:Kandarp Desai on LinkedInXactlyHow AI HappensSama
00:25:10
Sep 09, 2024
Srujana is Vice President and Group Director at Walmart’s Machine Learning Center of Excellence and is an experienced and respected AI, machine learning, and data science professional. She has a strong background in developing AI and machine learning models, with expertise in natural language processing, deep learning, and data-driven decision-making. Srujana has worked in various capacities in the tech industry, contributing to advancing AI technologies and their applications in solving complex problems. In our conversation, we unpack the trends shaping AI governance, the importance of consumer data protection, and the role of human-centered AI. Explore why upskilling the workforce is vital, the potential impact AI could have on white-collar jobs, and which roles AI cannot replace. We discuss the interplay between bias and transparency, the role of governments in creating AI development guardrails, and how the regulatory framework has evolved. Join us to learn about the essential considerations of deploying algorithms at scale, striking a balance between latency and accuracy, the pros and cons of generative AI, and more. Key Points From This Episode:Srujana breaks down the top concerns surrounding technology and data.Learn how AI can be utilized to drive innovation and economic growth.Navigating the adoption of AI with upskilling and workforce retention.The AI gaps that upskilling should focus on to avoid workforce displacement.Common misconceptions about biases in AI and how they can be mitigated. Why establishing regulations, laws, and policies is vital for ethical AI development.Outline of the nuances of creating an effective worldwide regulatory framework.She explains the challenges and opportunities of deploying algorithms at scale. Hear about the strategies for building architecture that can adapt to future changes. She shares her perspective on generative AI and what its best use cases are.Find out what area of AI Srujana is most excited about.Quotes:“By deploying [bias] algorithms we may be going ahead and causing some unintended consequences.” — @Srujanadev [0:03:11]“I think it is extremely important to have the right regulations and guardrails in place.” — @Srujanadev [0:11:32]“Just using generative AI for the sake of it is not necessarily a great idea.” — @Srujanadev [0:25:27]“I think there are a lot of applications in terms of how generative AI can be used but not everybody is seeing the return on investment.” — @Srujanadev [0:27:12]Links Mentioned in Today’s Episode:Srujana KaddevarmuthSrujana Kaddevarmuth on XSrujana Kaddevarmuth on LinkedInUnited Nations Association (UNA) San Francisco The World in 2050American INSIGHTHow AI HappensSama
00:31:13
Aug 29, 2024
Our guest goes on to share the different kinds of research they use for machine learning development before explaining why he is more conservative when it comes to driving generative AI use cases. He even shares some examples of generative use cases he feels are worthwhile. We hear about how these changes will benefit all UPS customers and how they avoid sharing private and non-compliant information with chatbots. Finally, Sunzay shares some advice for anyone wanting to become a leader in the tech world.Key Points From This Episode:Introducing Sunzay Passari to the show and how he landed his current role at UPS.Why Sunzay believes that this huge operation he’s part of will drive transformational change. How AI and machine learning have made their way into UPS over the past few years. The way Sunzay and his team have decided where AI will be most disruptive within UPS. Qualitative and qualitative research and what that looks like for this project. Why Sunzay is conservative when it comes to driving generative AI use cases. Sunzay shares some of the generative use cases that he thinks are worthwhile. The way these new technologies will benefit everyday UPS customers. How they are preventing people from accessing non-compliant data through chatbots. Sunzay passes on some advice for anyone looking to forge their career as a leader in tech. Quotes:“There’s a lot of complexities in the kind of global operations we are running on a day-to-day basis [at UPS].” — Sunzay Passari [0:04:35]“There is no magic wand – so it becomes very important for us to better our resources at the right time in the right initiative.” — Sunzay Passari [0:09:15]“Keep learning on a daily basis, keep experimenting and learning, and don’t be afraid of the failures.” — Sunzay Passari [0:22:48]Links Mentioned in Today’s Episode:Sunzay Passari on LinkedInUPSHow AI HappensSama
00:25:09
Aug 23, 2024
Martin shares what reinforcement learning does differently in executing complex tasks, overcoming feedback loops in reinforcement learning, the pitfalls of typical agent-based learning methods, and how being a robotic soccer champion exposed the value of deep learning. We unpack the advantages of deep learning over modeling agent approaches, how finding a solution can inspire a solution in an unrelated field, and why he is currently focusing on data efficiency. Gain insights into the trade-offs between exploration and exploitation, how Google DeepMind is leveraging large language models for data efficiency, the potential risk of using large language models, and much more. Key Points From This Episode:What it is like being a five times world robotic soccer champion.The process behind training a winning robotic soccer team.Why standard machine learning tools could not train his team effectively. Discover the challenges AI and machine learning are currently facing.Explore the various exciting use cases of reinforcement learning.Details about Google DeepMind and the role of him and his team. Learn about Google DeepMind’s overall mission and its current focus.Hear about the advantages of being a scientist in the AI industry. Martin explains the benefits of exploration to reinforcement learning.How data mining using large language models for training is implemented. Ways reinforcement learning will impact people in the tech industry.Unpack how AI will continue to disrupt industries and drive innovation.Quotes:“You really want to go all the way down to learn the direct connections to actions only via learning [for training AI].” — Martin Riedmiller [0:07:55]“I think engineers often work with analogies or things that they have learned from different [projects].” — Martin Riedmiller [0:11:16]“[With reinforcement learning], you are spending the precious real robots time only on things that you don’t know and not on the things you probably already know.” — Martin Riedmiller [0:17:04]“We have not achieved AGI (Artificial General Intelligence) until we have removed the human completely out of the loop.” — Martin Riedmiller [0:21:42]Links Mentioned in Today’s Episode:Martin RiedmillerMartin Riedmiller on LinkedInGoogle DeepMindRoboCupHow AI HappensSama
00:26:16